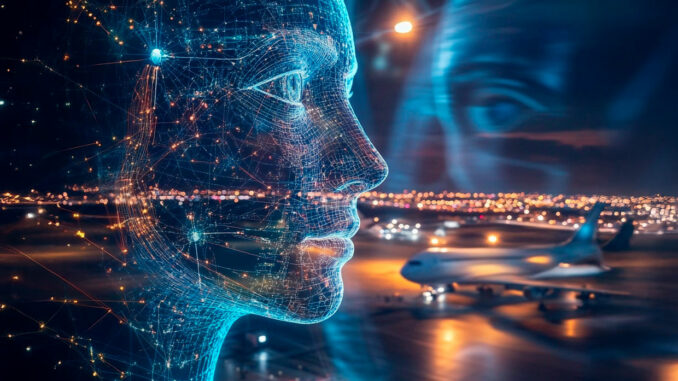
Artificial Intelligence (AI) is revolutionizing aviation by augmenting remote control capabilities for on-board aircraft systems. This article delves into the technical aspects and practical applications of AI in improving safety, efficiency, and reliability. The research focuses on AI-driven anomaly detection, predictive maintenance, real-time data analysis, and autonomous decision-making. Key AI technologies such as machine learning, deep learning, and natural language processing play pivotal roles in transforming the aviation sector.
The aviation industry is undergoing a significant shift towards automation and digitization. The complexity of modern aircraft systems generates vast data streams requiring efficient management. Traditional manual methods for remote control and monitoring have proven inadequate due to data volume and real-time response requirements. AI offers a pathway to address these challenges through advanced data analysis and decision-making tools.
Key Features of AI in Remote Control Systems
Real-Time Monitoring and Data Analysis in Aviation
Real-time monitoring and data analysis form the backbone of Artificial Intelligence (AI) applications in aviation. With aircraft generating terabytes of data per flight through sensors and embedded systems, traditional methods struggle to keep up with real-time requirements. AI addresses this challenge by processing, analyzing, and interpreting data at unparalleled speeds, ensuring efficient management and operational safety.
Key Components of Real-Time Monitoring
- Performance Metrics: AI systems continuously track critical performance parameters such as engine thrust, temperature, and pressure. This data is essential for maintaining optimal flight conditions.
- Sensor Outputs: Modern aircraft are equipped with thousands of sensors monitoring various subsystems, including hydraulics, avionics, and aerodynamics. AI integrates and analyzes these streams to provide a comprehensive operational picture.
- Environmental Conditions: External factors such as weather, air traffic, and terrain are dynamically analyzed by AI to ensure adaptive responses during flight.
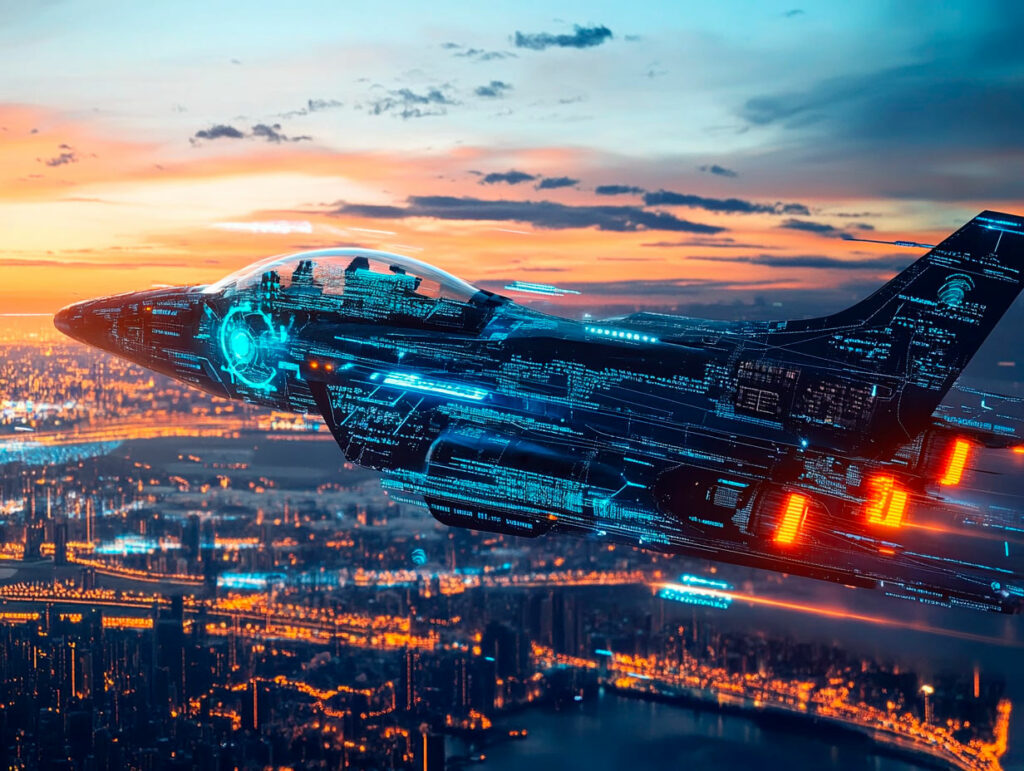
Machine Learning for Anomaly Detection
Machine learning (ML) models are trained on historical flight data to recognize normal operating patterns. By comparing real-time inputs against these benchmarks, the system detects deviations that could indicate potential problems, such as:
- Imminent mechanical failures.
- Abnormal fuel consumption patterns.
- Environmental hazards like sudden wind shear.
For example, if an engine sensor reports a slight but consistent increase in vibration beyond normal thresholds, the AI system flags this as a potential issue. This allows the ground crew to investigate and address the problem before it escalates.
Enhancing Decision-Making
By synthesizing vast datasets into actionable insights, AI aids ground control and flight operators in making prompt, informed decisions. Alerts and recommendations are prioritized based on severity, ensuring focus on critical issues.
Example: Fuel Efficiency Optimization
Fuel costs account for up to 30% of an airline’s operating expenses. AI monitors parameters such as engine RPM, airspeed, and throttle settings to identify suboptimal fuel usage. If inefficiencies are detected, such as improper engine calibration, the AI system suggests adjustments, reducing fuel consumption and greenhouse gas emissions.
Benefits of Real-Time Data Analysis
- Cost Reduction: Proactive identification of inefficiencies minimizes fuel usage and maintenance costs.
- Enhanced Safety: Early anomaly detection prevents in-flight failures, reducing the risk of accidents.
- Operational Efficiency: Streamlined decision-making processes improve overall system responsiveness.
By leveraging AI for real-time monitoring and analysis, aviation operators can ensure safer, more efficient, and cost-effective operations, setting a new standard for modern aircraft management.
Predictive Maintenance
Predictive maintenance (PdM) leverages AI technologies to transform how aircraft systems are monitored and maintained. Traditional maintenance practices rely on fixed schedules or reactive responses to failures, often resulting in unnecessary costs or unexpected downtime. Predictive maintenance, powered by AI, shifts this paradigm by forecasting potential issues based on real-time data and historical patterns.
Key Components of AI-Driven Predictive Maintenance
- Data Collection and Analysis:
- Aircraft systems generate massive amounts of operational data from sensors embedded in engines, hydraulics, avionics, and other critical subsystems.
- AI algorithms analyze this data to identify subtle patterns and trends that might precede a failure.
- Machine Learning Models:
- Trained on historical data, these models can recognize normal behavior and detect anomalies.
- For example, consistent deviations in vibration levels or fuel flow rates might indicate wear in engine components.
- Real-Time Insights:
- AI systems continuously monitor in-flight performance and provide real-time updates on system health.
- Alerts are prioritized based on severity, helping ground crews address the most critical issues first.
How Predictive Maintenance Works
- Step 1: Data Aggregation: Sensors collect performance metrics, such as engine temperature, oil pressure, and landing gear cycles.
- Step 2: Anomaly Detection: AI identifies deviations from normal operating conditions.
- Step 3: Failure Prediction: Based on historical trends, AI predicts the remaining useful life (RUL) of components.
- Step 4: Proactive Intervention: Maintenance teams receive actionable insights, allowing them to replace or repair components before failures occur.
Benefits of Predictive Maintenance
- Reduced Downtime:
- By addressing issues before they cause a failure, airlines minimize aircraft ground time.
- Studies reveal that predictive maintenance reduces downtime by up to 30%, enhancing fleet availability.
- Cost Savings:
- AI-driven insights prevent unnecessary part replacements and emergency repairs.
- Maintenance costs can decrease by approximately 20% due to optimized resource allocation.
- Improved Safety:
- Predictive systems enhance reliability by ensuring components are replaced or repaired before failure.
- This reduces in-flight emergencies and improves passenger confidence.
Example: Engine Monitoring Systems
Modern aircraft engines, such as those by Rolls-Royce or Pratt & Whitney, are equipped with advanced health monitoring systems. AI analyzes metrics like rotor speed, fuel efficiency, and temperature variations. If the system detects a gradual rise in exhaust gas temperature (EGT), it may indicate turbine wear. Maintenance teams can then schedule a replacement during planned downtime, avoiding costly in-flight engine failures.
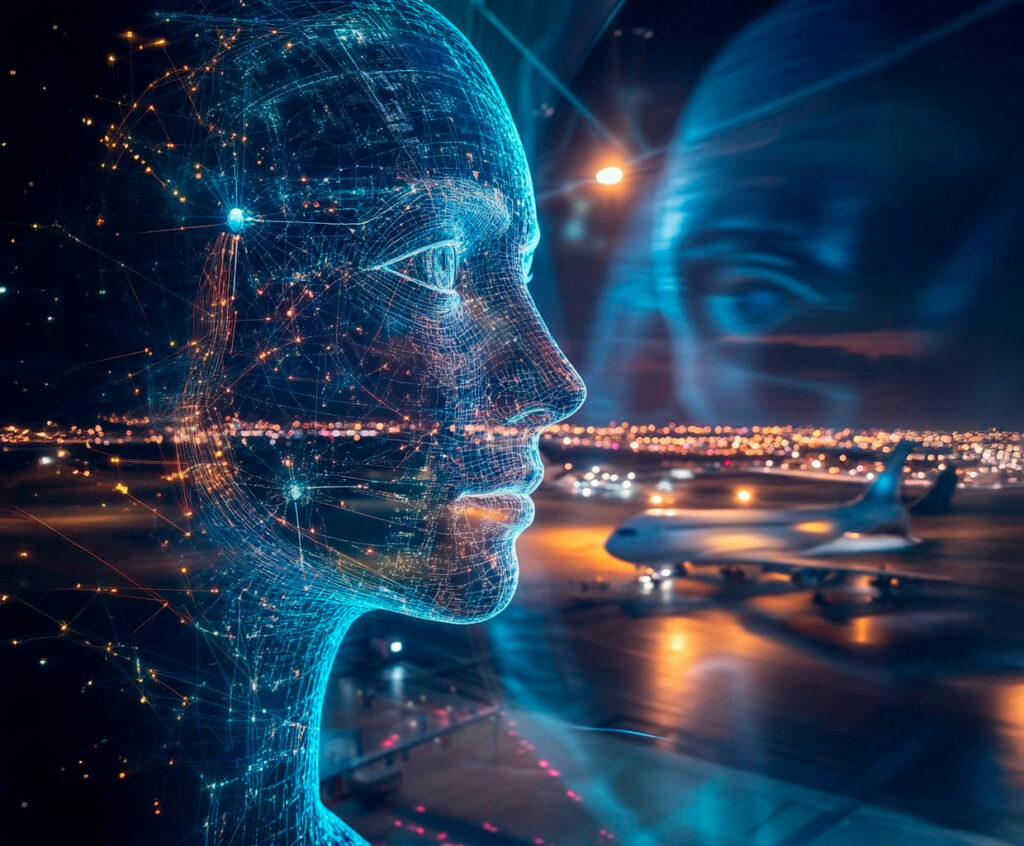
Challenges in Implementation
- Data Accuracy:
- Predictive models require high-quality, comprehensive datasets. Missing or incorrect data can reduce accuracy.
- Integration:
- Existing legacy systems in older aircraft may not fully support AI-driven predictive tools, necessitating costly upgrades.
Future of Predictive Maintenance in Aviation
- Edge Computing:
- Real-time processing at the edge (onboard the aircraft) reduces latency and enhances predictive accuracy.
- Collaborative Platforms:
- Airlines are adopting cloud-based predictive maintenance platforms that aggregate data across fleets to improve predictions.
- Market Growth:
- The global aviation maintenance market is expected to reach €80 billion by 2028, with predictive maintenance technologies driving a significant portion of this growth.
AI-driven predictive maintenance is reshaping aircraft operations by improving reliability, reducing costs, and ensuring safety. As technologies advance, its role in aviation will only expand, making it an indispensable tool for modern fleets.
Autonomous Decision-Making
Autonomous decision-making enabled by Artificial Intelligence (AI) represents a significant leap in modern aviation technology. It allows aircraft systems to process real-time data, evaluate multiple scenarios, and execute actions without direct human intervention. This capability reduces the cognitive load on pilots and ground operators, particularly during high-pressure or emergency situations.
Core Components of Autonomous Decision-Making
- Real-Time Data Processing:
- Aircraft sensors provide continuous streams of data, including airspeed, altitude, weather conditions, and system performance metrics.
- AI systems integrate this data to create a holistic picture of the aircraft’s current state.
- Multi-Variable Analysis:
- Advanced machine learning models assess numerous variables simultaneously to predict potential outcomes.
- For example, during a rapid loss of altitude, AI considers factors such as engine performance, external air pressure, and terrain to recommend or initiate corrective measures.
- Action Execution:
- Once a decision is reached, AI systems send commands to flight control systems or issue alerts to the crew, depending on the level of autonomy configured.
Applications of Autonomous Decision-Making
- Turbulence Management:
- AI systems detect unexpected turbulence through real-time sensor data.
- Adjustments are made to flight parameters such as altitude and speed, ensuring passenger comfort and reducing strain on the airframe.
- Emergency Handling:
- In critical scenarios like engine failure or hydraulic system malfunction, AI can autonomously reroute power, adjust flight paths, or initiate emergency landings.
- Example: AI systems in modern aircraft simulate glide paths for emergency landings, considering terrain, wind patterns, and available runways.
- Fuel Optimization:
- AI continuously monitors fuel usage and adjusts throttle settings to optimize consumption during flight.
- This not only reduces costs but also ensures sufficient fuel for contingencies.
Benefits of Autonomous Decision-Making
- Enhanced Safety:
- AI systems eliminate human error caused by fatigue or cognitive overload, providing consistent and precise responses.
- Autonomous systems can respond faster than human operators, crucial in time-sensitive situations.
- Operational Efficiency:
- Autonomous decision-making reduces pilot workload, enabling them to focus on strategic decision-making rather than routine tasks.
- Example: AI manages inflight cabin pressurization adjustments, freeing up crew resources.
- Improved Reliability:
- AI systems continuously learn from historical and real-time data, refining their decision-making processes over time.
Example: Airbus Autonomous Taxiing and Flight Path Adjustment
Airbus has tested autonomous systems capable of handling taxiing, takeoff, and landing. These systems use AI to navigate runways, avoid obstacles, and align with flight paths, even in low-visibility conditions. During unexpected events, such as abrupt wind shifts during landing, AI recalculates the descent trajectory to ensure a safe touchdown.
Challenges and Limitations
- Complex Decision Scenarios:
- Not all scenarios can be anticipated or pre-programmed. AI systems may struggle with ambiguous or novel situations requiring human judgment.
- Regulatory Hurdles:
- Autonomous decision-making systems must comply with stringent aviation regulations, which can slow their adoption.
- System Reliability:
- AI systems must operate with near-zero failure rates to gain widespread trust from operators and passengers.
Future Outlook
- Hybrid Systems:
- Many modern aircraft employ a hybrid approach where AI assists but does not replace human operators. This provides a balance of efficiency and accountability.
- Integration with Autonomous Air Traffic Control:
- AI-driven aircraft will eventually collaborate with autonomous air traffic control systems, further reducing delays and optimizing routes.
- Market Potential:
- The autonomous aviation market, including AI systems, is projected to grow to €25 billion by 2035, driven by advances in AI and increasing demand for efficient air travel.
AI-driven autonomous decision-making is redefining the aviation landscape, enhancing safety and efficiency while reducing human dependency in critical operations. Its continued development will pave the way for smarter, safer, and more efficient skies.
Challenges in Implementing AI in Aviation Systems
The implementation of AI in aviation, particularly for remote control systems, presents several technical and operational challenges. Among the most significant are energy requirements and data security and privacy, which directly impact system reliability, scalability, and adoption.
Energy Requirements
AI technologies rely on computationally intensive algorithms, such as machine learning and deep learning, which demand substantial processing power. This poses several challenges for aircraft systems:
1. High Computational Power Needs
- AI systems require powerful hardware, including GPUs (Graphics Processing Units) and specialized processors like TPUs (Tensor Processing Units).
- These components consume significant amounts of energy, which is a critical limitation for aviation, where every kilogram of equipment and every watt of power impacts operational efficiency.
2. Aircraft Energy Constraints
- Aircraft are designed with strict energy budgets to power essential systems, including avionics, navigation, and communication. Adding energy-intensive AI hardware strains these limits.
- For instance, AI systems for predictive maintenance or anomaly detection must process data continuously, increasing power consumption during flight.
3. Solutions Under Development
- Advances in edge computing allow data to be processed locally on the aircraft, reducing reliance on energy-intensive cloud processing. However, edge devices still require optimization for energy efficiency.
- Emerging technologies like quantum computing and low-power AI chips aim to address these challenges. For example, low-power processors by NVIDIA and Intel are being adapted for aviation applications, potentially reducing energy consumption by up to 40%.
Data Security and Privacy
AI systems in aviation depend on the seamless exchange of vast amounts of sensitive data between aircraft and ground control systems. This raises critical concerns about data security and privacy.
1. Risks in Communication Channels
- Remote control systems rely on satellite communication (SATCOM) and high-frequency radio links to exchange data. These channels are vulnerable to interception, hacking, and spoofing.
- A cyberattack compromising AI systems could disrupt operations, lead to false commands, or expose sensitive flight data.
2. Compliance with Standards
- AI solutions must adhere to stringent aviation regulations, such as those set by the European Union Aviation Safety Agency (EASA) or the Federal Aviation Administration (FAA).
- Compliance involves encryption standards, secure software development practices, and robust data storage protocols to protect sensitive information.
3. Privacy Concerns
- AI systems often collect and process personal data from passengers and crew, such as biometric information for access controls. Ensuring compliance with privacy regulations like the General Data Protection Regulation (GDPR) is critical.
4. Mitigation Strategies
- Implementation of end-to-end encryption for all data transmissions minimizes the risk of interception.
- AI systems employ intrusion detection algorithms to identify unauthorized access attempts in real-time.
- The development of blockchain-based frameworks ensures tamper-proof data integrity, which is particularly useful for maintaining secure aircraft logs.
Combined Impact of These Challenges
The combined effects of energy requirements and data security concerns increase the complexity of implementing AI-driven remote control systems. These challenges often require trade-offs between system performance and operational feasibility:
- Increased Costs:
- High-performance AI systems and cybersecurity measures significantly raise development and operational costs. For example, integrating AI into existing systems may require upgrades to hardware, software, and infrastructure, costing airlines millions of euros per fleet.
- Delayed Adoption:
- Smaller airlines or organizations with limited budgets may delay adopting AI technologies until more cost-effective and energy-efficient solutions are developed.
- Operational Risks:
- If these challenges are not adequately addressed, the reliability of AI systems could be compromised, potentially jeopardizing flight safety.
Future Prospects
- Energy Solutions:
- Research into renewable energy sources for aircraft, such as fuel cells or solar-assisted systems, could offset the additional energy burden of AI systems.
- Efficient power management algorithms designed specifically for aviation are expected to optimize energy allocation, ensuring critical systems remain unaffected.
- Advanced Cybersecurity Measures:
- The integration of quantum cryptography offers the potential to create nearly unbreakable encryption methods, ensuring secure communication even against sophisticated cyberattacks.
- AI can be leveraged to protect itself, with autonomous systems detecting and mitigating security threats in real-time.
- Collaborative Development:
- Partnerships between aviation authorities, AI developers, and cybersecurity experts are essential to address these challenges holistically. Collaborative frameworks will ensure that new technologies meet both operational and regulatory requirements.
By addressing energy demands and enhancing data security, AI systems will become more feasible and reliable for widespread adoption in aviation, paving the way for smarter, safer, and more efficient aircraft operations.
The integration of AI in aviation transforms aircraft operations, offering unparalleled advantages in efficiency, safety, and reliability. Continued research and development in AI technologies will further streamline remote control systems, paving the way for smarter and safer aviation.
War Wings Daily is an independant magazine.